Overview
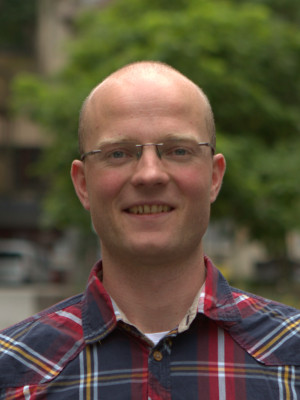
Welcome! My name is Philipp Geiger, on this web page I give an overview over my work in research and software applications, and general background. I'm a research scientist at Bosch Center for Artificial Intelligence. Before, I did my doctorate in computer science and a postdoc at Max Planck Institute for Intelligent Systems and University of Stuttgart, including a stay at Microsoft Research Cambridge, and my diplom in mathematics with philosophy as side subject at Heidelberg University and Humboldt University of Berlin.
Generally, I conduct research and development in the areas of machine learning (core part of artificial intelligence) and multiagent systems (analysis and optimization of multiple intelligent decision makers' interaction behavior). Often, this combines project-driven software engineering of machine learning pipelines (currently mainly Python/PyTorch) and experimental aspects with statistical/mathematical aspects. With this, I aim to contribute to the analysis and solution of application problems that are important to business and society as a whole. Currently, I am focusing on multiagent imitation learning and reinforcement learning often combined with aspects of deep learning and generative models, with application (proof of concept and protoypes) to data-driven driving behavior models, autonomous driving and its safety validation (see also further background).
Selected publications (see also all publications and further material):
- Fail-Safe Adversarial Generative Imitation Learning. (2022). TMLR.
- Learning game-theoretic models of multiagent trajectories using implicit layers. (2021). AAAI.
- Coordinating users of shared facilities via data-driven predictive assistants and game theory. (2019). UAI. [Slides.]
Further links: my profiles on Google Scholar, DBLP, OpenReview, GitHub.