Overview
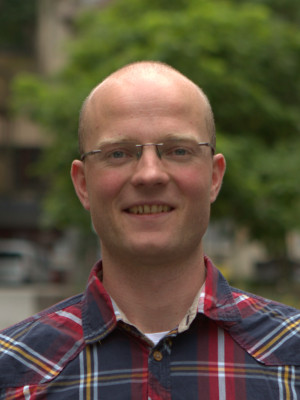
Welcome! My name is Philipp Geiger, on this web page I give an overview over my research and background. I'm a research scientist at Bosch Center for Artificial Intelligence. Before, I did my doctorate in computer science and a postdoc at Max Planck Institute for Intelligent Systems and University of Stuttgart, including a stay at Microsoft Research Cambridge, and my diplom in mathematics with philosophy as side subject at Heidelberg University and Humboldt University of Berlin.
Generally, I conduct research in the areas of machine learning (core part of artificial intelligence) and multiagent systems (analysis and optimization of multiple intelligent decision makers' interaction behavior). Often, this combines mathematical/theoretical aspects with software engineering and experimental aspects. With this, I aim to contribute to the analysis and solution of application problems that are important to business and society as a whole. Currently, I am focusing on deep generative imitation learning and data-driven realistic multiagent behavior simulations, with application to autonomous driving and its safety validation (see also further background).
Selected publications (see also all publications and further material):
- Fail-Safe Adversarial Generative Imitation Learning. (2022). TMLR.
- Learning game-theoretic models of multiagent trajectories using implicit layers. (2021). AAAI.
- Coordinating users of shared facilities via data-driven predictive assistants and game theory. (2019). UAI. [Slides.]
Further links: my profiles on Google Scholar, DBLP, OpenReview, GitHub.